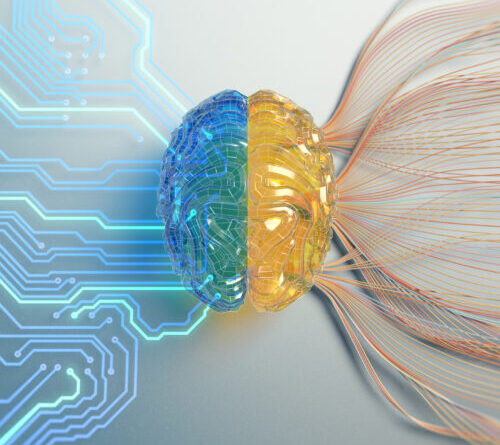
In the scientists’ COCONUT design (for Chain Of CONtinUous Thought), those sort of concealed states are encoded as “latent thoughts” that change the private written actions in a rational series both throughout training and when processing a question. This prevents the requirement to transform to and from natural language for each action and “frees the reasoning from being within the language space,” the scientists compose, causing an enhanced thinking course that they describe a “continuous thought.”
Being more breadth-minded
While doing rational processing in the hidden area has some advantages for design performance, the more crucial finding is that this type of design can “encode multiple potential next steps simultaneously.” Instead of needing to pursue specific sensible choices completely and one by one (in a “greedy” sort of procedure), remaining in the “latent space” permits a type of instantaneous backtracking that the scientists compare to a breadth-first-search through a chart.
This emergent, synchronised processing home comes through in screening although the design isn’t clearly trained to do so, the scientists compose. “While the model may not initially make the correct decision, it can maintain many possible options within the continuous thoughts and progressively eliminate incorrect paths through reasoning, guided by some implicit value functions,” they compose.
A figure highlighting a few of the methods various designs can stop working at specific kinds of sensible reasoning.
Credit: Training Large Language Models to Reason in a Continuous Latent Space
That type of multi-path thinking didn’t truly enhance COCONUT’s precision over conventional chain-of-thought designs on fairly simple tests of mathematics thinking (GSM8K) or basic thinking (ProntoQA). The scientists discovered the design did relatively well on an arbitrarily produced set of ProntoQA-style questions including complex and winding sets of sensible conditions (e.g., “every apple is a fruit, every fruit is food, etc.”.
For these jobs, basic chain-of-thought thinking designs would typically get stuck down dead-end courses of reasoning and even hallucinate totally fabricated guidelines when attempting to fix the rational chain. Previous research study has actually likewise revealed that the “verbalized” sensible actions output by these chain-of-thought designs “may actually utilize a different latent reasoning process” than the one being shared.
This brand-new research study signs up with a growing body of research study looking for to comprehend and make use of the method big language designs operate at the level of their underlying neural networks. And while that sort of research study hasn’t resulted in a big advancement right now, the scientists conclude that designs pre-trained with these type of “continuous thoughts” from the start might “enable models to generalize more effectively across a wider range of reasoning scenarios.”
Learn more
As an Amazon Associate I earn from qualifying purchases.