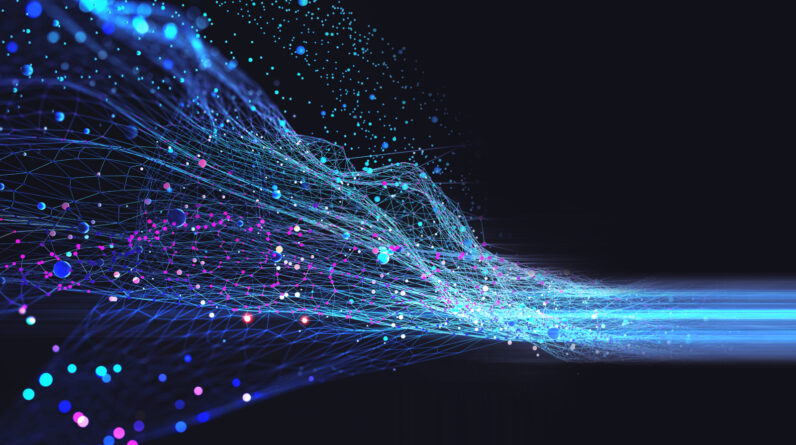
Neural networks that underpin LLMs may not be as clever as they appear.
( Image credit: Yurchanka Siarhei/Shutterstock)
Generative expert system (AI)systems might have the ability to produce some mind-blowing outcomes however brand-new research study reveals they do not have a meaningful understanding of the world and genuine guidelines.
In a brand-new research study released to the arXiv preprint database, researchers with MIT, Harvard and Cornell discovered that the big language designs (LLMs ), like GPT-4 or Anthropic’s Claude 3 Opusstop working to produce underlying designs that properly represent the real life.
When charged with supplying turn-by-turn driving instructions in New York City, for instance, LLMs provided them with near-100 % precision. The underlying maps utilized were complete of non-existent streets and paths when the researchers extracted them.
The scientists discovered that when unanticipated modifications were contributed to an instruction(such as detours and closed streets), the precision of instructions the LLMs provided plunged. Sometimes, it led to overall failure. It raises issues that AI systems released in a real-world circumstance, state in a driverless automobile, might malfunction when provided with vibrant environments or jobs.
Related: AI’can stunt the abilities required for independent self-creation’: Relying on algorithms might improve your whole identity without you understanding
“One hope is that, because LLMs can accomplish all these amazing things in language, maybe we could use these same tools in other parts of science, as well. But the question of whether LLMs are learning coherent world models is very important if we want to use these techniques to make new discoveries,” stated senior author Ashesh Rambachanassistant teacher of economics and a primary detective in the MIT Laboratory for Information and Decision Systems (LIDS), in a declaration
Challenging transformers
The essence of generative AIs is based upon the capability of LLMs to gain from huge quantities of information and specifications in parallel. In order to do this they depend on transformer designswhich are the underlying set of neural networks that process information and make it possible for the self-learning element of LLMs. This procedure produces a so-called “world model” which a skilled LLM can then utilize to presume responses and produce outputs to inquiries and jobs.
Get the world’s most interesting discoveries provided directly to your inbox.
One such theoretical usage of world designs would be taking information from taxi journeys throughout a city to create a map without requiring to fastidiously outline every path, as is needed by present navigation tools. If that map isn’t precise, discrepancies made to a path would trigger AI-based navigation to underperform or stop working.
To evaluate the precision and coherence of transformer LLMs when it pertains to comprehending real-world guidelines and environments, the scientists evaluated them utilizing a class of issues called deterministic limited automations (DFAs). These are issues with a series of states such as guidelines of a video game or crossways in a path en route to a location. In this case, the scientists utilized DFAs drawn from the parlor game Othello and navigation through the streets of New York.
To evaluate the transformers with DFAs, the scientists took a look at 2 metrics. The very first was “sequence determination,” which examines if a transformer LLM has actually formed a meaningful world design if it saw 2 various states of the very same thing: 2 Othello boards or one map of a city with roadway closures and another without. The 2nd metric was “sequence compression” — a series (in this case a purchased list of information points utilized to produce outputs) which need to reveal that an LLM with a meaningful world design can comprehend that 2 similar states, (state 2 Othello boards that are precisely the exact same) have the very same series of possible actions to follow.
Depending on LLMs is danger
2 typical classes of LLMs were evaluated on these metrics. One was trained on information created from arbitrarily produced series while the other on information created by following tactical procedures.
Transformers trained on random information formed a more precise world design, the researchers discovered, This was perhaps due to the LLM seeing a larger range of possible actions. Lead author Keyon Vafaa scientist at Harvard, discussed in a declaration: “In Othello, if you see two random computers playing rather than championship players, in theory you’d see the full set of possible moves, even the bad moves championship players wouldn’t make.” By seeing more of the possible relocations, even if they’re bad, the LLMs were in theory much better prepared to adjust to random modifications.
In spite of creating legitimate Othello relocations and precise instructions, just one transformer created a meaningful world design for Othello, and neither type produced a precise map of New York. When the scientists presented things like detours, all the navigation designs utilized by the LLMs stopped working.
“I was surprised by how quickly the performance deteriorated as soon as we added a detour. If we close just 1 percent of the possible streets, accuracy immediately plummets from nearly 100 percent to just 67 percent,” included Vafa.
This reveals that various methods to using LLMs are required to produce precise world designs, the scientists stated. What these techniques might be isn’t clear, however it does highlight the fragility of transformer LLMs when confronted with vibrant environments.
“Often, we see these models do impressive things and think they must have understood something about the world,” concluded Rambachan. “I hope we can convince people that this is a question to think very carefully about, and we don’t have to rely on our own intuitions to answer it.”
Roland Moore-Colyer is a self-employed author for Live Science and handling editor at customer tech publication TechRadar, running the Mobile Computing vertical. At TechRadar, among the U.K. and U.S.’ biggest customer innovation sites, he concentrates on smart devices and tablets. Beyond that, he taps into more than a years of composing experience to bring individuals stories that cover electrical cars (EVs), the advancement and useful usage of synthetic intelligence (AI), combined truth items and utilize cases, and the development of calculating both on a macro level and from a customer angle.
Many Popular
Find out more
As an Amazon Associate I earn from qualifying purchases.