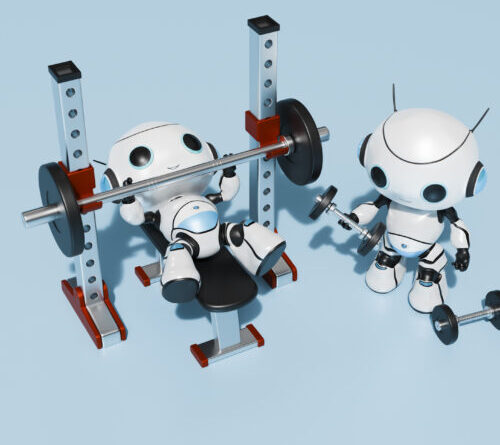
Does size matter?
Memory requirements are the most apparent benefit of minimizing the intricacy of a design’s internal weights. The BitNet b1.58 design can run utilizing simply 0.4 GB of memory, compared to anywhere from 2 to 5GB for other open-weight designs of approximately the very same specification size.
The streamlined weighting system likewise leads to more effective operation at reasoning time, with internal operations that rely much more on easy addition directions and less on computationally pricey reproduction directions. Those performance enhancements imply BitNet b1.58 utilizes anywhere from 85 to 96 percent less energy compared to comparable full-precision designs, the scientists quote.
A demonstration of BitNet b1.58 performing at speed on an Apple M2 CPU.
By utilizing an extremely enhanced kernel created particularly for the BitNet architecture, the BitNet b1.58 design can likewise run several times much faster than comparable designs working on a basic full-precision transformer. The system is effective enough to reach “speeds comparable to human reading (5-7 tokens per second)” utilizing a single CPU, the scientists compose(you can download and run those enhanced kernels yourself on a variety of ARM and x86 CPUs, or attempt it utilizing this web demonstration).
Most importantly, the scientists state these enhancements do not come at the expense of efficiency on different standards evaluating thinking, mathematics, and “knowledge” abilities (although that claim has yet to be confirmed individually). Balancing the outcomes on numerous typical standards, the scientists discovered that BitNet “achieves capabilities nearly on par with leading models in its size class while offering dramatically improved efficiency.”
In spite of its smaller sized memory footprint, BitNet still carries out likewise to “full precision” weighted designs on lots of criteria.
In spite of its smaller sized memory footprint, BitNet still carries out likewise to “full precision” weighted designs on lots of criteria.
Regardless of the obvious success of this “proof of concept” BitNet design, the scientists compose that they do not rather comprehend why the design works along with it makes with such streamlined weighting. “Delving deeper into the theoretical underpinnings of why 1-bit training at scale is effective remains an open area,” they compose. And more research study is still required to get these BitNet designs to take on the general size and context window “memory” these days’s biggest designs.
Still, this brand-new research study reveals a prospective alternative method for AI designs that are dealing with spiraling hardware and energy expenses from working on costly and effective GPUs. It’s possible that today’s “full precision” designs resemble muscle vehicles that are losing a great deal of energy and effort when the equivalent of a great sub-compact might provide comparable outcomes.
Find out more
As an Amazon Associate I earn from qualifying purchases.