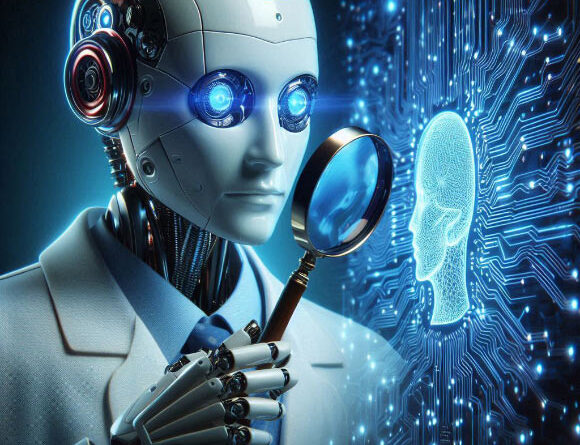
ChatGPT and other large language models (LLMs), comprising billions of parameters and pre-trained on extensive web-scale corpora, have been claimed to acquire certain capabilities without having been specifically trained on them. These capabilities, referred to as emergent abilities, have been a driving force in discussions regarding the potentials and risks of language models. In their new paper, University of Bath researcher Harish Tayyar Madabushi and colleagues present a new theory that explains emergent abilities, taking into account their potential confounding factors, and rigorously substantiate this theory through over 1,000 experiments. Their findings suggest that purported emergent abilities are not truly emergent, but result from a combination of in-context learning, model memory, and linguistic knowledge.
Lu et al. suggest large language models like ChatGPT cannot learn independently or acquire new skills.
“The prevailing narrative that this type of AI is a threat to humanity prevents the widespread adoption and development of these technologies, and also diverts attention from the genuine issues that require our focus,” Dr. Tayyar Madabushi said.
Dr. Tayyar Madabushi and colleagues ran experiments to test the ability of LLMs to complete tasks that models have never come across before — the so-called emergent abilities.
As an illustration, LLMs can answer questions about social situations without ever having been explicitly trained or programmed to do so.
While previous research suggested this was a product of models ‘knowing’ about social situations, the researchers showed that it was in fact the result of models using a well-known ability of LLMs to complete tasks based on a few examples presented to them, known as ‘in-context learning’ (ICL).
Through thousands of experiments, the team demonstrated that a combination of LLMs ability to follow instructions, memory and linguistic proficiency can account for both the capabilities and limitations exhibited by LLMs.
“The fear has been that as models get bigger and bigger, they will be able to solve new problems that we cannot currently predict, which poses the threat that these larger models might acquire hazardous abilities including reasoning and planning,” Dr. Tayyar Madabushi said.
“This has triggered a lot of discussion — for instance, at the AI Safety Summit last year at Bletchley Park, for which we were asked for comment — but our study shows that the fear that a model will go away and do something completely unexpected, innovative and potentially dangerous is not valid.”
“Concerns over the existential threat posed by LLMs are not restricted to non-experts and have been expressed by some of the top AI researchers across the world.”
However, Dr. Tayyar Madabushi and co-authors maintain this fear is unfounded as their tests clearly demonstrated the absence of emergent complex reasoning abilities in LLMs.
“While it’s important to address the existing potential for the misuse of AI, such as the creation of fake news and the heightened risk of fraud, it would be premature to enact regulations based on perceived existential threats,” Dr. Tayyar Madabushi said.
“Importantly, what this means for end users is that relying on LLMs to interpret and perform complex tasks which require complex reasoning without explicit instruction is likely to be a mistake.”
“Instead, users are likely to benefit from explicitly specifying what they require models to do and providing examples where possible for all but the simplest of tasks.”
“Our results do not mean that AI is not a threat at all,” said Technical University of Darmstadt’s Professor Iryna Gurevych.
“Rather, we show that the purported emergence of complex thinking skills associated with specific threats is not supported by evidence and that we can control the learning process of LLMs very well after all.”
“Future research should therefore focus on other risks posed by the models, such as their potential to be used to generate fake news.”
_____
Sheng Lu et al. 2024. Are Emergent Abilities in Large Language Models just In-Context Learning? arXiv: 2309.01809
As an Amazon Associate I earn from qualifying purchases.