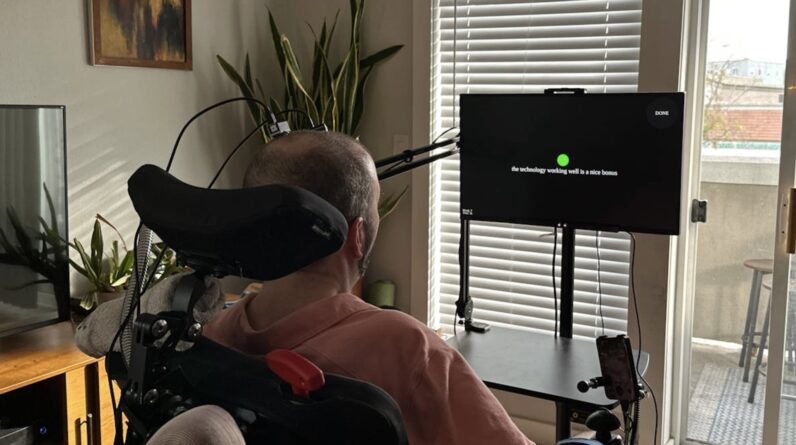
(Image credit: Nicholas Card)
Brain-computer user interfaces are a cutting-edge innovation that can assist paralyzed individuals gain back functions they’ve lost, like moving a hand. These gadgets record signals from the brain and analyze the user’s desired action, bypassing harmed or broken down nerves that would typically send those brain signals to manage muscles.
Because 2006presentations of brain-computer user interfaces in people have actually mainly concentrated on bring back arm and hand motions by making it possible for individuals to control computer system cursors or robotic armsJust recently, scientists have actually started establishing speech brain-computer user interfaces to bring back interaction for individuals who can not speak.
As the user tries to talk, these brain-computer user interfaces tape-record the individual’s special brain signals connected with tried muscle motions for speaking and after that equate them into words. These words can then be shown as text on a screen or spoken aloud utilizing text-to-speech software application.
I’m a reseacher in the Neuroprosthetics Lab at the University of California, Davis, which belongs to the BrainGate2 scientific trial. My associates and I just recently showed a speech brain-computer user interface that analyzes the tried speech of a male with ALS, or amyotrophic lateral sclerosis, likewise called Lou Gehrig’s illness. The user interface transforms neural signals into text with over 97% precision. Secret to our system is a set of expert system language designs– synthetic neural networks that assist translate natural ones.
Related: New ‘thought-controlled’ gadget checks out brain activity through the jugular
Recording brain signals
The primary step in our speech brain-computer user interface is taping brain signals. There are numerous sources of brain signals, a few of which need surgical treatment to record. Surgically implanted recording gadgets can catch premium brain signals since they are put closer to nerve cells, leading to more powerful signals with less disturbance. These neural recording gadgets consist of grids of electrodes put on the brain’s surface area or electrodes implanted straight into brain tissue.
In our research study, we utilized electrode ranges surgically positioned in the speech motor cortex, the part of the brain that manages muscles associated with speech, of the individual, Casey Harrell. We tape-recorded neural activity from 256 electrodes as Harrell tried to speak.
Deciphering brain signals
The next difficulty is relating the complex brain signals to the words the user is attempting to state.
One technique is to map neural activity patterns straight to spoken words. This technique needs tape-recording brain signals representing each word numerous times to determine the typical relationship in between neural activity and particular words. While this method works well for little vocabularies, as shown in a 2021 research study with a 50-word vocabularyit ends up being unwise for bigger ones. Think of asking the brain-computer user interface user to attempt to state every word in the dictionary numerous times– it might take months, and it still would not work for brand-new words.
Rather, we utilize an alternative method: mapping brain signals to phonemes, the fundamental systems of noise that comprise words. In English, there are 39 phonemes, consisting of ch, er, oo, pl and sh, that can be integrated to form any word. We can determine the neural activity related to every phoneme several times simply by asking the individual to check out a couple of sentences aloud. By precisely mapping neural activity to phonemes, we can assemble them into any English word, even ones the system wasn’t clearly trained with.
To map brain signals to phonemes, we utilize sophisticated maker finding out designs. These designs are especially appropriate for this job due to their capability to discover patterns in big quantities of complicated information that would be difficult for human beings to determine. Think about these designs as super-smart listeners that can select crucial details from loud brain signals, just like you may concentrate on a discussion in a congested space. Utilizing these designs, we had the ability to analyze phoneme series throughout tried speech with over 90% precision.
From phonemes to words
As soon as we have actually the analyzed phoneme series, we require to transform them into words and sentences. This is difficult, particularly if the analyzed phoneme series isn’t completely precise. To fix this puzzle, we utilize 2 complementary kinds of artificial intelligence language designs.
The very first is n-gram language designs, which forecast which word is more than likely to follow a set of n words. We trained a 5-gram, or five-word, language design on countless sentences to forecast the probability of a word based upon the previous 4 words, catching regional context and typical expressions. After “I am extremely excellent,” it may recommend “today” as more most likely than “potato”. Utilizing this design, we transform our phoneme series into the 100 more than likely word series, each with an associated likelihood.
The 2nd is big language designs, which power AI chatbots and likewise forecast which words probably follow others. We utilize big language designs to fine-tune our options. These designs, trained on large quantities of varied text, have a more comprehensive understanding of language structure and significance. They assist us identify which of our 100 prospect sentences makes one of the most sense in a broader context.
By thoroughly stabilizing possibilities from the n-gram design, the big language design and our preliminary phoneme forecasts, we can make an extremely informed guess about what the brain-computer user interface user is attempting to state. This multistep procedure permits us to deal with the unpredictabilities in phoneme decoding and produce meaningful, contextually suitable sentences.
Real-world advantages
In practice, this speech decoding method has actually been extremely effective. We’ve made it possible for Casey Harrell, a guy with ALS, to “speak” with over 97% precision utilizing simply his ideas. This advancement permits him to quickly speak with his friends and family for the very first time in years, all in the convenience of his own home.
Speech brain-computer user interfaces represent a substantial advance in bring back interaction. As we continue to improve these gadgets, they hold the pledge of providing a voice to those who have actually lost the capability to speak, reconnecting them with their liked ones and the world around them.
Difficulties stay, such as making the innovation more available, portable and resilient over years of usage. In spite of these obstacles, speech brain-computer user interfaces are an effective example of how science and innovation can come together to resolve intricate issues and drastically enhance individuals’s lives.
This edited short article is republished from The Conversation under a Creative Commons license. Check out the initial post
As an Amazon Associate I earn from qualifying purchases.